Revolutionizing Risk Prediction and Damage Prevention with Data and AI
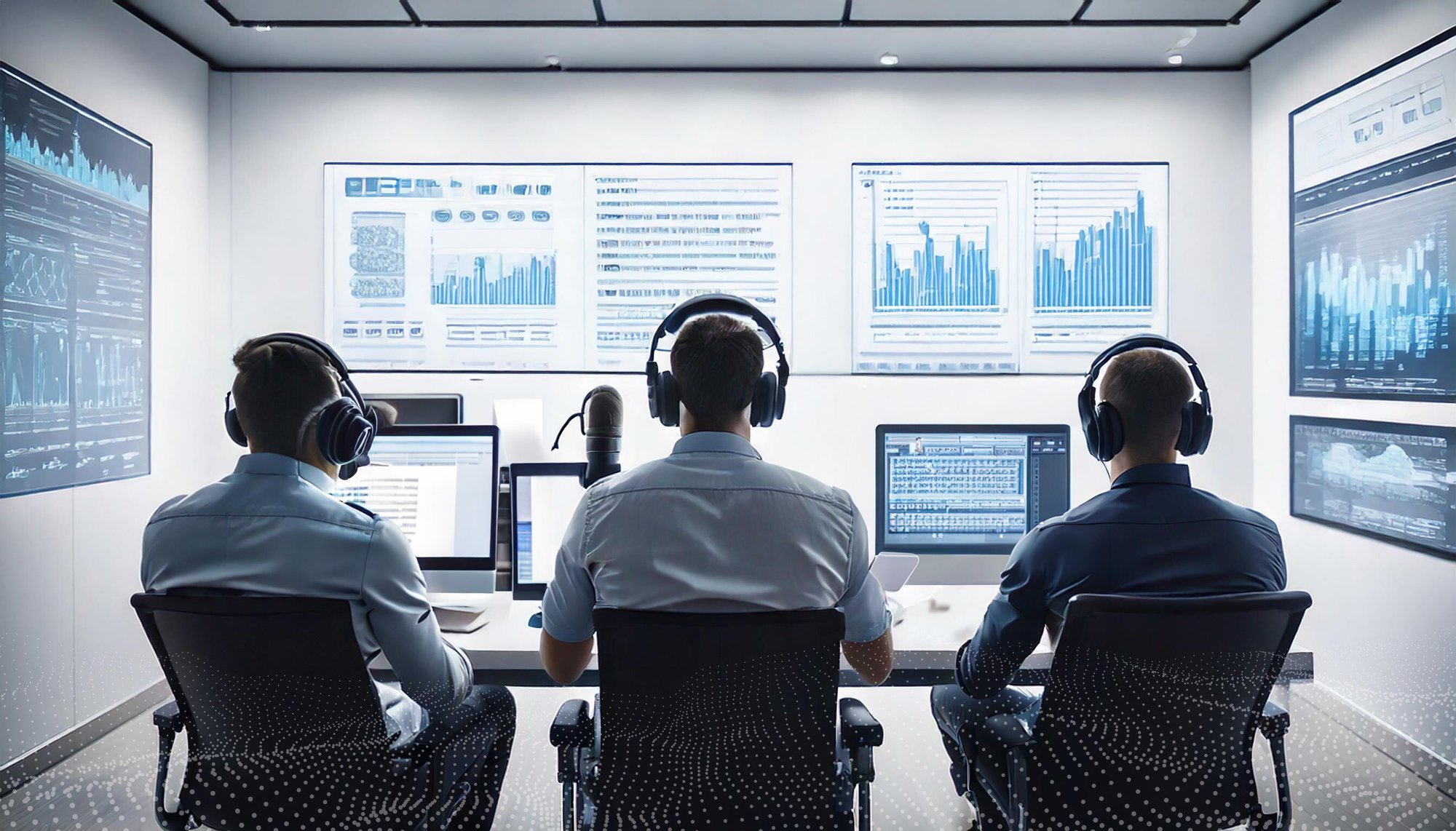
When data scientists dive into the data that companies create in day-to-day operations, they seek to find ways for those companies to do what they do just a little bit better.
On the Irth podcast, host Jason Adams talked with Zank Bennett, CEO of Bennett Data Science, and Kyle Murphy, Irth’s Director of Data and Architecture, about how they began to explore data and use artificial intelligence (AI) to uncover valuable damage prevention insights. What started as a proof of concept in 2019 with a little effort that yielded tremendous results has become a commitment to revolutionize risk prediction and damage prevention by extracting further insights from the data.
How AI identifies and solves complex problems
Before AI can extract risk and damage prevention insights from data, it must be trained. This is when data scientists teach an algorithm to recognize patterns.
To illustrate, consider a spreadsheet with many columns. These columns contain the details of what you know and what has happened at a dig site. For example, excavator, type of asset, weather conditions, etc. In the last column, there is either a one to denote an incident occurred or a zero if it didn’t.
Typically, data scientists prefer a data set to have 50% ones and 50% zeroes for the most efficient algorithm training. Algorithm training requires positive and negative results to learn from. Thankfully, there are a lot fewer ones (meaning an incident) than zeroes. However, this reality presented an interesting challenge when Irth was training data sets, making the extraordinary results achieved with current models even more impressive.
The more data you have, the better — millions of lines on our imaginary spreadsheet.
Data scientists create a training data set with 80% of that data by giving the algorithm all the data known except for whether there was an incident. The data scientists then assess the model’s performance. Maybe the first time, the algorithm successfully identifies an incident 30% of the time and gets it wrong 70% of the time. Training continues, and the model is fine-tuned until it meets accuracy thresholds.
We use supervised learning to guide machines to learn most effectively. This allows SMEs to inject their knowledge into the training process when appropriate.
Why should you trust AI insights?
AI isn’t magic. It’s pattern recognition that’s backed by advanced statistics and probability.
With interpretability, when you get a prediction, you have an idea of what went into that prediction. In some industries, such as insurance, providing interpretability is required.
Irth added interpretability models in 2023. This allows our customers to see the why behind insights and understand the patterns through explainability factors.
While some might refer to data scientists as wizards, there’s math behind all machine learning and artificial intelligence. This isn’t a black-box model where there isn’t a reason for the predictions. We can say it works and show why it works.
Descriptive, predictive, and prescriptive analytics
Descriptive analytics look at what has already happened. This data is often reported on dashboards. It’s looking back to report the data, but it’s not “smart” in any way.
When we talk about risk factors, we use predictive analytics. Predictive analytics takes historical and real-time data and applies statistical analysis to determine what might happen in the future. It can even tell us why — the explainability factors.
The next frontier is prescriptive analytics. In damage prevention, our goal is to tell our customers where damage will be, where it will be if you intervene, and what you should do based on probability. It’s about putting a “probability cloud” around incidents, Bennett explained during the podcast.
AI can unearth counterintuitive facts
Damage prevention experts with decades of experience understand risk factors. They know in their guts what will likely result in an incident because they’ve seen it during their careers. It’s critical to talk to subject matter experts to determine what’s important to them and to learn how AI can add to that knowledge.
AI thrives on more data and continuously learns from new information. It can surface risk factors that might even seem counterintuitive to those experts, but the data shows that they are more likely to be present when an incident occurs.
Currently, Irth has a North American model that gives us an incredible perspective on mitigating risk. When a customer’s data is added to the model, the insights can get even more precise and become prescriptive.
AI models require drift monitoring
Every time there is an intervention, it changes the game a bit. Interventions can be anything from sending a notification to say we’ve identified a riskier area to rolling out a truck to the site for a watch and protect.
You change the baseline as you continue to intervene consistently in these high-probability risks. This is known as drift. New laws, regulations, methods, and best practices create changes over time, which inevitably cause drift. Data scientists must monitor models to detect drift and then take appropriate action, whether that’s fixing data quality issues or retraining the model to ensure accuracy over time.
Irth trains our models as often as necessary to ensure they are as accurate and representative of recent history as possible. If an incident occurs, our customers can rest assured that the models learned from that, and tomorrow’s predictions will account for that new learning.
Even if you do nothing, 10 years from now, your predictions will be significantly less relevant than they are today. We live in an ever-changing world, so data scientists must constantly consider past, present, and future events. This is the only way you’ll continue getting value from AI.
Damage prevention possibilities
Irth receives 70% of all 811 tickets in the industry. This represents hundreds of millions of tickets with as many as 70 fields of data on every ticket. Today, we’re using this data pool, in addition to environmental, training comprehension, and other data, to predict incident likelihood, incident impact, and whether on-site field visits are appropriate. We are actively working toward providing new and exciting predictive insights in the months to come!