Risk Management for Utilities: Combining Damage Risk Analysis and Artificial Intelligence
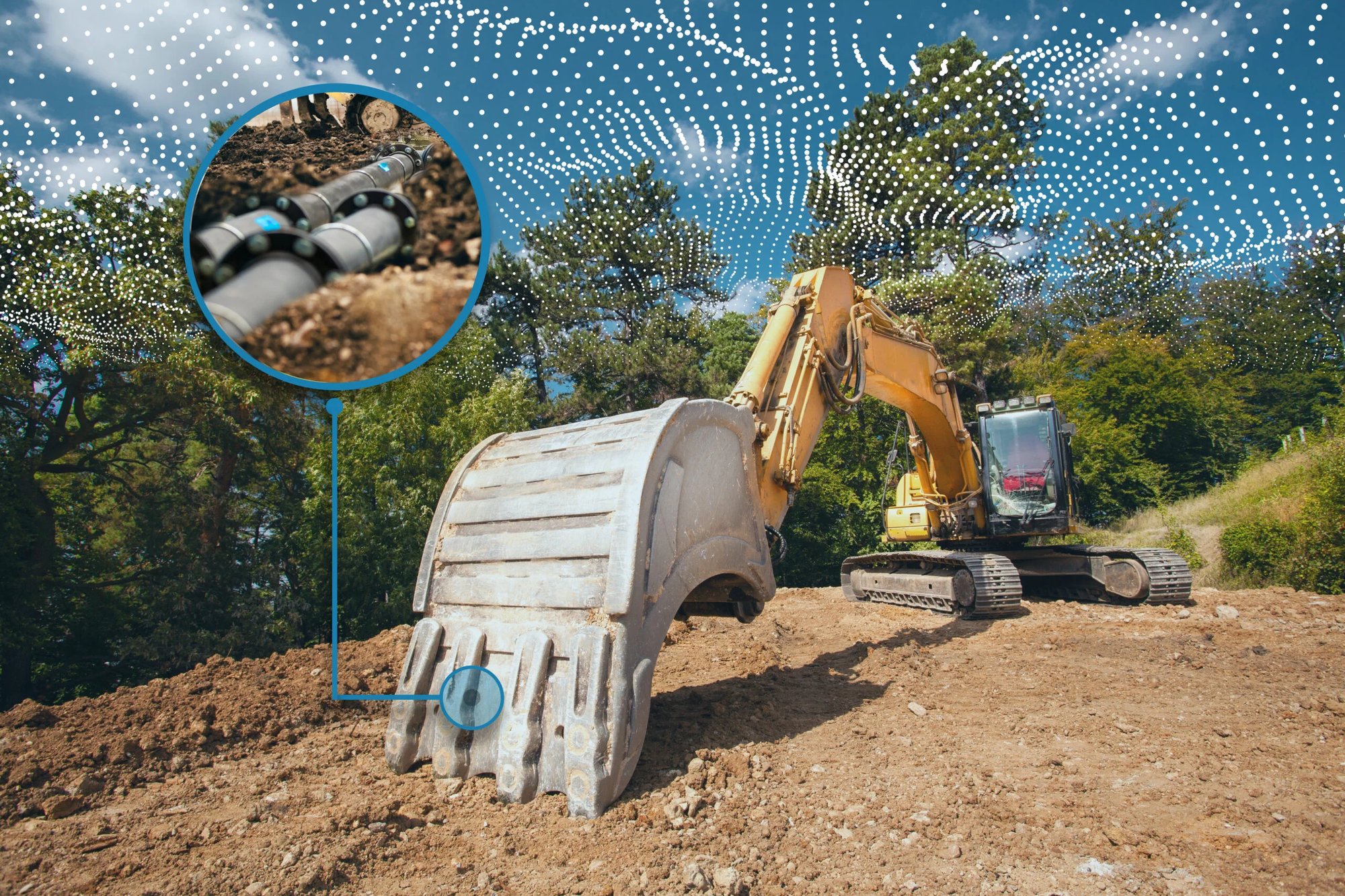
Risk management for utilities requires a dual approach in the digital era: historical and predictive data analytics.
As underground critical network infrastructure expands, it’s essential to use all data available to score risk.
Pacific Gas & Electric (PG&E) committed to burying 10,000 miles of power lines in the next decade to prevent fires from igniting from its equipment. Telecoms are burying thousands of miles of high-speed fiber optic cable for enhanced internet and network services.
Coinciding with this expansion is additional construction activity propelled by the Infrastructure Investment and Jobs Act that commits $1.2 trillion to new infrastructure projects. These projects include roads, bridges, power and grid, broadband, water infrastructure, ports/waterways, public transit, passenger and freight rail, and more. This construction will happen near existing underground utilities and naturally increases risk.
Dig tickets are on the rise. Damages are increasing. And less experienced locators are replacing those with years of experience. This creates the perfect storm for damage to critical network infrastructure.
This situation requires a new approach to risk management for utilities. One that not only looks at what transpired in the past but uses artificial intelligence to predict what could happen in the future. Using historical (or “actuarial”) and predictive models to assess risk, utilities are better positioned to manage risk efficiently and effectively to prevent damage and service outages.
A Historical Model of Risk Management for Utilities
It’s impossible to oversee every mile of infrastructure or every activity near a critical asset. Therefore, energy companies, utilities, telecoms, and governments prioritize efforts on tickets that pose the biggest risk to assets.
Utilities typically assess risk by looking at locator, excavator, and asset data. By looking at damage data that occurred in the past, Damage Risk Analysis automatically assesses the risks of activities and proposes the next best action to mitigate risk. This might include creating a locate audit before a dig occurs.
By using Risk Scoring, high-risk dig tickets can be prioritized.
When you think about this model of risk management for utilities, it operates similarly to auto insurance. If you’re a driver with multiple traffic tickets, accidents, or claims, the insurance company sees you at higher risk for future claims because your history shows that you are more likely to continue that pattern.
In risk management for utilities, if an excavator or locator has a history of near misses or damages, they receive a higher risk score. Their history on tickets is assessed to determine how risky they are on any new tickets associated with them.
A Predictive Model of Risk Management for Utilities Using Artificial Intelligence (AI)
One of the benefits of using artificial intelligence (AI) to power risk management for utilities is that AI can reveal complex relationships and patterns in the data set. A predictive risk management model uses damage data to predict what is likely to occur.
Our Insights with AI uses each customer’s data sets as well as anonymized data from one call tickets and 811 ticket centers.
In one analysis of the top 10% of tickets using anonymized data from 811 call centers, we were able to catch 52% of damages. When using client-specific data sets, we found 53% of damages. This is powerful insight for organizations that don’t have useable damage data of their own.
Historical Model + Predictive Model = Comprehensive Risk Management for Utilities
Irth risk management for utilities is the only solution that combines damage risk analysis that leverages historical and predictive models for the most comprehensive assessment of risk.
Past damage activity doesn’t always equate to future damages. However, predictive is not always accurate either. A combination of both models gives the most depth to risk scoring.
The predictive AI risk model allows historical models to become more dynamic and responsive to future changes. When the AI model identifies potential trends, our platform applies the results in the field immediately. The AI predictive risk model applies this trend analysis and updates the model to use the trend data and historical data to identify risk. As you experience fewer damages in the field, the risk factors also change. As these changes occur, the historical model becomes more flexible in identifying risk on targeted factors.
Even after using our system for more depth in risk management, how can you confirm that you’re doing your best to reduce the risks that have been identified?
With Irth’s training and assessment platform powered by Coursettra, you can train field personnel and verify the field changes are having positive effects. With our training and operator qualification, you can target training for high-risk areas to keep workers safer.
With threats continuing to multiply and critical network infrastructure expanding, utilities require a two-prong approach to risk management that not only looks at what occurred in the past but also forecasts what might occur in the future with an AI assessment of data from multiple sources.
This solution is only available through Irth. Contact us today to get a demo of our risk management for utilities.